2016 Recipients of C&C Prize
Group B
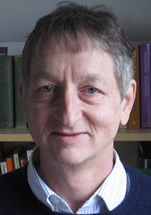
Prof. Geoffrey E. Hinton
Emeritus Professor, University of Toronto
Distinguished Researcher, Google Inc.
Citation
For Outstanding Contributions to Neural network Research and the Pioneering Development of Innovative Deep-learning Technology
Achievement
Artificial intelligence (AI) is a term that refers to the use of computers to achieve human-like intelligence, such as learning, reasoning, and judgment. AI research began in the mid-1950s, and there were high expectations regarding its application to the industrial sector. The neural network approach to AI is inspired by the way the brain performs computation, and neural networks underwent research booms in the 1960s and 1980s. However, owing to limited computing power, limited data, and technical hurdles, neural networks did not achieve widespread practical use. Instead, the research booms were followed by research winters.
Professor Geoffrey E. Hinton is the foremost contributor to the establishment of neural network technology. The deep-learning technology that he developed was a breakthrough in the field of machine learning. It accelerated the practical application of machine learning to industrial fields, becoming the driving force in the development of artificial intelligence as it exists today. Prof. Hinton’s research on deep learning has had a tremendous impact on speech-recognition technology and computer vision. It led to large improvements in speech recognition, image analysis, medical diagnosis, computer games, and enhanced vehicle safety, bringing about a revolution in machine learning and its applications.
Neural network technology utilizes computational models inspired by the neural circuitry of the brain. Prof. Hinton has numerous achievements to his credit in the field of neural networks, starting with the second AI boom in the 1980s. First, in 1983, jointly with Prof. Terry Sejnowski, he proposed the Boltzmann machine, a kind of neural network that operates stochastically and has a simple, local learning rule for creating complex internal representations. This allowed neural networks to invent their own feature detectors. Then, in 1985, Prof. Hinton collaborated with Prof. David Rumelhart and Prof. Ronald Williams and demonstrated the effectiveness of the backpropagation algorithm as a learning method for multilayer neural networks. This is what led to the second AI boom.
However, the training procedure for Boltzmann machines originally proposed by Prof. Hinton and Prof. Sejnowski required too much computation to be practical. In 2002, Prof. Hinton overcame this obstacle by discovering an efficient training procedure called “contrastive divergence”. It was for Boltzmann machines with a restriction on their connectivity. The restriction was first proposed by Prof. Paul Smolensky in 1986, and with the efficient new training procedure, these restricted Boltzmann machines (RBMs) then became widely used.
In a seminal paper in 2006, Prof. Hinton et al. showed that RBMs could be stacked, with each new RBM treating the representations learned by the previous RBM as its data. This allowed deep multilayer networks to be “pre-trained” one layer at a time without requiring any labeled data. It also solved a long-standing problem of finding an efficient inference procedure for densely connected graphical models. In 2009, stacked RBMs were used to achieve dramatic improvements in speech recognition. This ushered in the era of deep learning.
In 2010, a competition called the ImageNet Large Scale Visual Recognition Challenge (ILSVRC) began. In it, researchers vied for the lowest error rate for recognizing a thousand different types of objects in natural images. In the 2012 competition, Prof. Hinton’s team used deep convolutional neural networks to achieve a 15% error rate, a major improvement over the 25% error rate achieved by the best other team. This result had a big impact on a lot of researchers in the machine-learning field. In the wake of this demonstration, deep learning was widely recognized as an innovative technology for achieving breakthroughs in the area of object recognition. Many researchers and companies then began research in earnest on deep learning and its industrial applications.
By using multiple layers of neurons, deep learning allows a neural network to extract a hierarchy of complex features that greatly facilitates accurate and robust pattern recognition. The basic concepts and techniques have been known since the 1980s, but three factors were required to make them successful at an industrial scale. The first factor was advances in the types of neurons and their training procedures. These advances included techniques introduced by Prof. Hinton, such as rectified linear neurons and “dropout” regularization. The second factor was large increases in the amount of data available for training. The third and most important factor was the explosive growth in computing power, which has enabled the processing of ever-greater amounts of data by ever-larger neural networks. As a result of these three factors, deep learning has already been widely deployed in speech recognition and image interpretation and is rapidly moving toward deployment in other commercial applications such as video understanding, machine translation, drug design, health care, and robotics.
Deep learning has shown that computers can automatically extract the features required for recognition in the process of learning, something that was beyond the capability of earlier approaches. In particular, neural networks can now learn how to represent the meanings of words and of whole sentences as large vectors of neural activity. This is truly revolutionary, making it possible to simulate human thought processes, including conceptualizing the characteristics of things and using these conceptualizations for intuitive reasoning.
The neural network approach to artificial intelligence is now experiencing its third boom following those of the 1960s and 1980s. The combination of powerful deep-learning algorithms, advances in big-data processing technology, and huge increases in computing power have brought us to a stage where artificial intelligence is capable of taking on previously impossible challenges. It is safe to say that we are about to enter a period of full-fledged application and popularization. Artificial intelligence is a fundamental technology that is essential for the provision of efficient and sophisticated services and the resolution of increasingly complex social challenges. Going forward, it is expected to develop into a technology with enormous potential for energizing society through its application to many different industries.
As described above, Prof. Hinton’s research on neural networks has revolutionized the field of artificial intelligence. Through his pioneering roles, he has made major contributions not only to the development of information and communications technology, but also to socio-economic and industrial development. This is a record of achievement that fully deserves the C&C Prize.